Trivedi receives grant funding from DOE, NSF to develop hardware tools
Trivedi receives grant funding from DOE, NSF to develop hardware tools Heading link
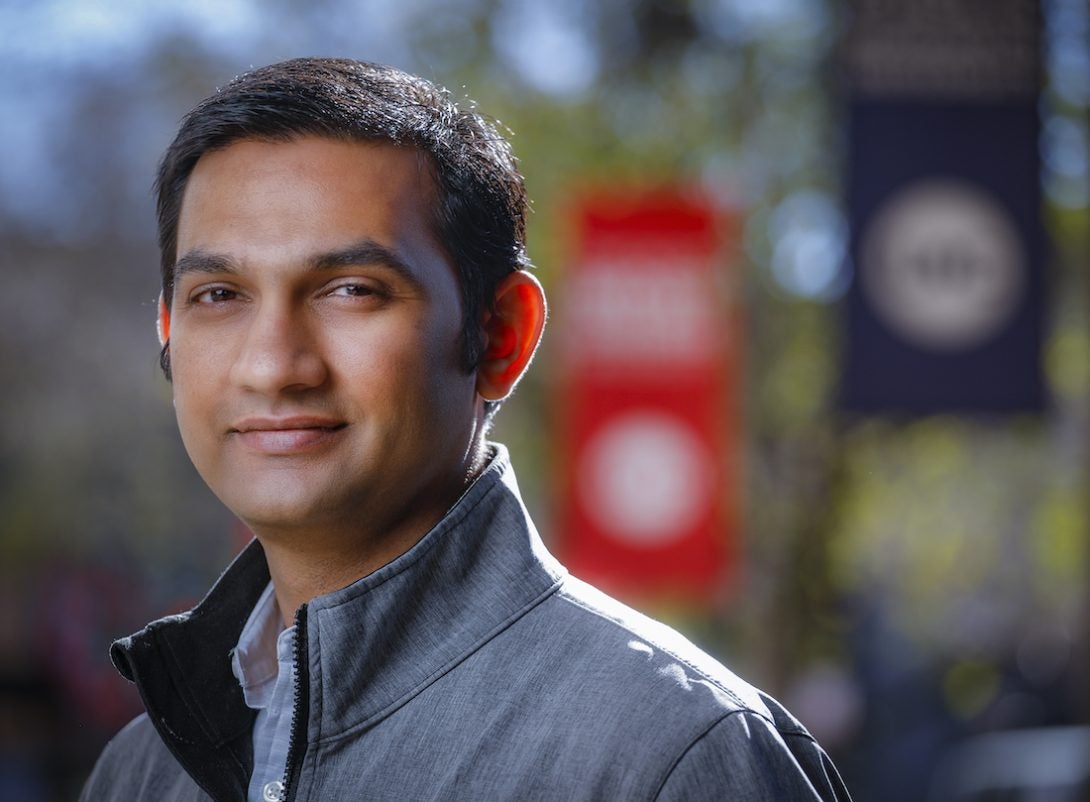
Associate Professor Amit R. Trivedi has received four grants in the last twelve months, focused on a variety of computer hardware tools and applications. Trivedi’s current work touches on physics, robotics, and machine learning.
Trivedi has 11 parallel grants, with more than three million dollars in current research funding.
Beyond the Higgs Boson Heading link
The discovery of the Higgs Boson a decade ago completed the standard model of particle physics, but many fundamental questions about the Universe remain. CERN, the international organization that operates the world’s largest particle physics laboratory, is working on the High Luminosity Large Hadron Collider project, scheduled to come online in 2027. But the volume of data generated by this project will be massive, increasing data volume by a factor of ten from what the Large Hadron Collider has been collecting.
Trivedi’s part of the project, Pixel-Integrated Neural Processing for Extreme Precision Readout of Collision Events, is focused on developing a chip or hardware solution that sits with the experiment, and quickly processes through the transfer data being collected to identify the less than one percent of useful data for subsequent processing.
“Hundreds of terabytes of information are being generated every 14 nanoseconds, so we can save only the part of the data that will be useful, that identifies interesting events,” Trivedi said. “That information can be stored and analyzed later by physicists.”
Trivedi (PI) is collaborating with ECE’s Professor Ahmet Enis Cetin and Associate Professor Corrine Mills from the physics department on this $450,000 Department of Energy grant.
He gratefully acknowledges the support he received from Fermi National Accelerator Laboratory and Discovery Partners Institute for pursuing this grant.
Emulating the cerebellum Heading link
Robotics relies on the data-driven learning of deep neural networks (DNN), which greatly simplifies the complexity of decision-making problems. But DNNs are like black boxes, with no guarantees of accurate predictions. To assess when DNN predictions are inaccurate, large amounts of data must be processed. Extracting that information within the time and energy limits of edge devices isn’t feasible with current semiconductors.
Semiconductors are used to build devices such as switches that turn off and on, or that work with zeros and ones. Trivedi and a team of researchers are designing new types of semiconductors, those that can work with complex, random numbers and variables. They are designing a system that can emulate the mechanism of the cerebellum in a human brain.
Our cerebellum, a tiny area in the rear portion of our brain, helps us coordinate motor functions. This powerhouse holds half the neurons in our brain and helps us immediately correct our footing on changing terrain or snap out of deep concentration if we hear our name called in a loud, busy space.
“The goal of this project is to develop something similar to the cerebellum,” Trivedi said. “These chips can listen to massive amounts of data, very easily identifying what is unique or suspicious, just like a human brain.”
The NSF grant, FuSe-TG: Ultra-low-power and Robust Autonomy of Edge Robotics with 2D Semiconductors, includes Trivedi (PI), Sawyer Fuller at the University of Washington, and Mark Hersam and Vinod Sangwan from Northwestern University as co-PIs. UIC’s Theja Tulabandhula is the senior person on the grant. UIC’s share is $130,000.
Continuous learning isn’t just for humans Heading link
Continual learning is key to survival for humans and other creatures. For autonomous robots, the same is true; it is essential for their functionality when deployed in the field.
“A lot of machine learning models and hardware being developed are typically trained in the cloud, and once you start using them, they don’t learn anymore,” Trivedi said. “But the world isn’t static. The models don’t stay accurate.”
The researchers are developing a new transistor that follows a similar mechanism to what occurs in the human brain. Rather than using electrons like a typical transistor, these transistors will work based on the movement of ions, replicating what happens in synapses and neurons in a human.
“We are building a framework for localization mapping so robots can identify themselves in space, and account for changes in the space, updating their awareness of the environment around them,” Trivedi said.
This will also allow the personalization of a device to a particular user. Currently, items such as Fitbits or other smart devices are common for everyone, diminishing the utilization of the item.
The NSF grant, Co-designing Continual-Learning Edge Architectures with Hetero-Integrated Silicon-CMOS and Electrochemical Random-Access Memory, includes UIUC’s Qing Cao (PI), Saugata Ghose (co-PI), and Shaloo Rakheja (Co-PI); and Curtis Shoaf of Parkland College (co-PI). UIC’s share of the nearly two-million-dollar grant is $393,564.
Open-source hardware for science discovery Heading link
Machine learning has revolutionized scientific discovery in disciplines such as biology, climate research, astronomy, and physics. The success of machine learning algorithms is affected by latency, interference, energy efficiency, and reliability, but to date, limited attention has been paid to the deployment of machine learning hardware tools.
“We are trying to find solutions where we can have more democratized chips. On the software side, much is open source, and anyone can access them,” Trivedi said. “To develop hardware-based solutions, a lot of these chips are under a lot of IP and NDA constraints, so these solutions are not available.”
The NSF grant, POSE: Phase II: An Open Source Ecosystem for Collaborative Rapid Design of Edge AI Hardware Accelerators for Integrated Data Analysis and Discovery, includes Seda Memik (PI) from Northwestern University), Sule Ozen (Co-PI) from Arizona State University), Mark Neubauer (Co-PI) from UIUC, Amit Ranjan Trivedi (Co-PI), and Ahmet Enis Cetin (Co-PI) from UIC, and Jagadeesh Yedetore from Discovery Partners Institute. UIC’s share of the grant is $171,572, shared equally between Trivedi and Cetin.
He gratefully acknowledges the support he received from Fermi National Accelerator Laboratory and Discovery Partners Institute for pursuing this grant.