Trivedi, Shylendra develop a more realistic spiking neural network
Trivedi, Shylendra develop a more realistic spiking neural network Heading link
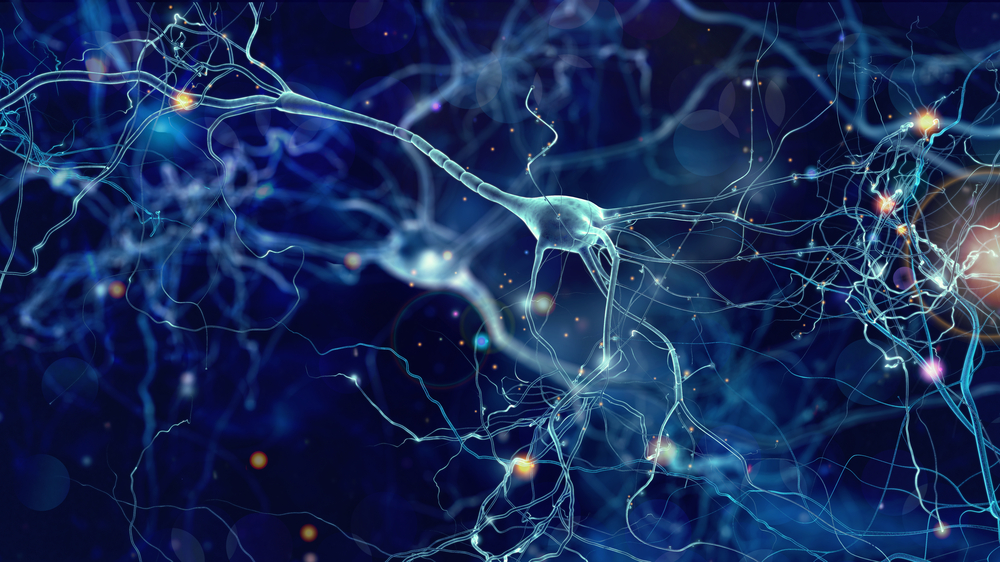
Assistant professor Amit Ranjan Trivedi and Ahish Shylendra, a third-year PhD student in Trivedi’s Advanced Electronics of Nano-devices (AEON) Lab, have developed a more efficient way to operate artificial neural networks. By incorporating a novel new nanomaterial, they have created a spiking neural network that more closely resembles the way a real brain operates.
Artificial neural networks, the systems used by computers to “learn” to recognize objects, faces, and all manner of data, are inspired by our brains—just as we learn by recognizing and processing information we take in, neural networks are trained, or taught, with data sets and refined so their accuracy improves.
But unlike a human brain, where spatial and temporal dimensions are seamlessly intertwined to process our sensory inputs, most artificial neural networks are rather simplistic. For example, a biological brain will coalesce time and space dimensions of an input scene and process both dimensions simultaneously, whereas an artificial neural network will split an input video in frames and process that same scene one frame at a time.
The result of this sequential processing is that an artificial neural network will exert about the same amount of computing effort on input frames where not much is happening, a process that Trivedi describes as wasteful. A biological brain is sensitive to events, and our neurons only fire when something “interesting” happens around us.
Spiking neural networks better resemble the way a brain operates: neuron activity is driven by events in the environment, and only sporadic spikes or bursts need to be interchanged among neurons, even when processing a very complex situation. Creating these types of artificial networks can be more difficult to train but more closely mimic biology and are far more energy efficient.
“The human brain is one thing that works so well. It has evolved over millennia and is the best artificial intelligence machine nature could design for us, so it’s good to coopt,” Trivedi said. “How the human brain works is an enigma, and we’re trying to solve it from multiple angles.”
Using a novel one-atom thick material developed by Northwestern Professor Mark Hersam and the Hersam Group at Northwestern, a team that develops nanoscale materials for computing applications, Trivedi and Shylendra were able to more closely replicate the behavior of a neuron in a human brain. The artificial neuron designed in the collaborative study showed variety of spiking modes that are found in a biological neuron, such as constant spiking, phasic spiking, phasic bursting, tonic bursting, spike latency, integrator response, dampened tonic bursting, and Class-I spiking.
“There are different modes of spiking for different sensors in the eyes or ears, for example, as well as different cognitive mechanisms. Achieving all these different modes of spiking in a compact structure will help us model the behavior of a biological brain more efficiently and enhance our learning model,” Shylendra said.
This model differs from the approach most researchers are using to incorporate into spiking neural networks and is far less complex. It is small, using seven or eight nanoscale components, which can be connected. It will be scalable to integrate millions of neurons in a compact computing chip and will consume far less power than most artificial intelligence accelerator chips available today. Trivedi said they worked with one neuron very efficiently and are trying to connect and disconnect neurons in their network to understand how a brain actually functions.
“A human brain takes 20 watts of power and can do a lot of things. An artificial intelligence system will take hundreds of watts of power to only do a tiny proportion of those things. We’re trying to find a better way to do things than today,” Trivedi said.
Trivedi is mostly working on AI and machine learning systems, from the hardware and implementation side. His research vision is to enable AI even in the tiniest electronic system, such as sensor nodes, so that these systems begin to act like biological beings and have more natural interactions with people.
The research paper, “Spiking neurons from tunable Gaussian heterojunction transistors,” was published earlier this year in Nature Communications.