Seferoglu, Koyuncu build testbed for edge computing systems
Seferoglu, Koyuncu build testbed for edge computing systems
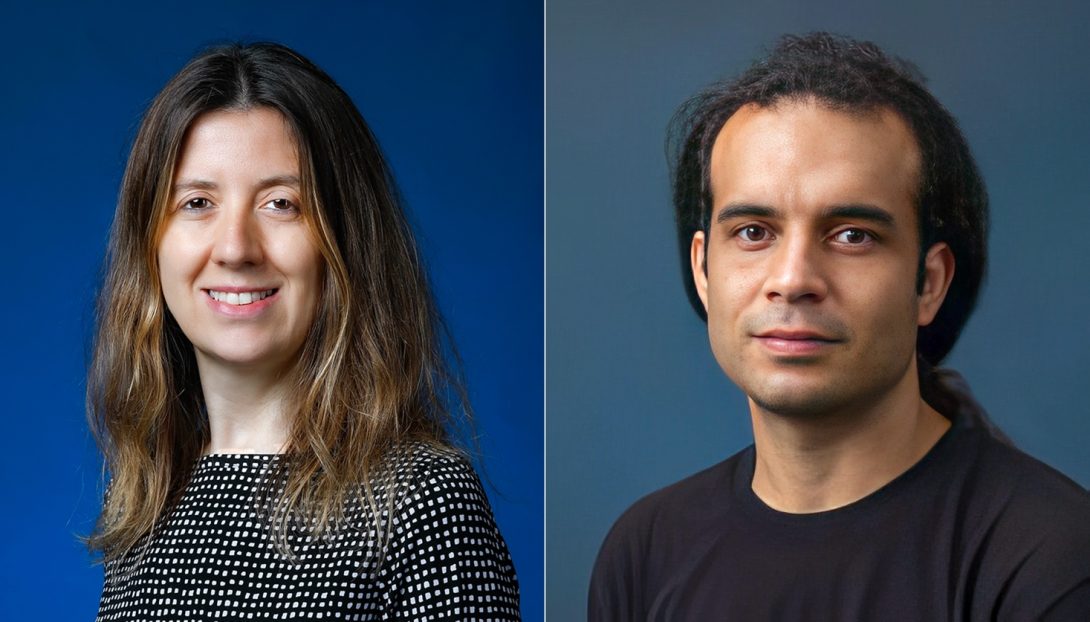
Associate Professor Hulya Seferoglu and Assistant Professor Erdem Koyuncu have been awarded $152,470 from the Army Research Office to improve the speed, accuracy, and security of Machine Learning (ML) computing during army missions.
ML and artificial intelligence (AI) algorithms use significant resources, from memory to computing power. Typically, these computation-intensive tasks are sent to the cloud for processing. But in the field, this central processing may not be available, or it may not be safe, so a machine learning model can be split into tasks and calculations that are distributed to nearby edge devices. These devices include computer servers and computers, cell phones, and other handheld devices.
“Machine learning models can be large, and they are getting larger with language models, so it doesn’t fit on one device,” Seferoglu said. “These edge devices often face constraints like limited computing, memory, storage resources, and potential network disruptions, especially since they are mobile, distributed, intermittent, and disconnected.”
Protecting the privacy of the data being processed by these edge devices is critical.
“We design methods to provide privacy of this data, and to ensure these calculations are done correctly and protect against devices that are behaving maliciously,” Seferoglu said.
To simulate field conditions using edge devices in resource-constrained environments, Seferoglu and Koyuncu are building a testbed that will emulate these systems on a smaller scale. The equipment will facilitate testing novel ML algorithms and optimization protocols, and provide feedback to AI/ML theory, helping refine models to better reflect the behavior of tactical edge computing systems.
The grant, Resource-Constrained Tactical Edge Computing System for Artificial Intelligence and Machine Learning, builds on a previous award to improve the performance of edge devices through ML algorithms and AI.